2018-11-28
阅读量:
1020
梯度下降的python实现
假设我们(以某种方式)为参数 theta_0 设定了某个初始值,那么可以如下使用梯
度下降法:
def minimize_batch(target_fn, gradient_fn, theta_0, tolerance=0.000001):
"""use gradient descent to find theta that minimizes target function"""
step_sizes = [100, 10, 1, 0.1, 0.01, 0.001, 0.0001, 0.00001]
theta = theta_0 # 设定theta为初始值
target_fn = safe(target_fn) # target_fn的安全版
value = target_fn(theta) # 我们试图最小化的值
while True:
gradient = gradient_fn(theta)
next_thetas = [step(theta, gradient, -step_size)
for step_size in step_sizes]
# 选择一个使残差函数最小的值
next_theta = min(next_thetas, key=target_fn)
next_value = target_fn(next_theta)
# 当“收敛”时停止
if abs(value - next_value) < tolerance:
92 | 第 8 章
return theta
else:
theta, value = next_theta, next_value
我们称它为 minimize_batch,因为在每一步梯度计算中,它都会搜索整个数据集(因为
target_fn 代表整个数据集的残差)。在下一部分中,我们会探讨另一种方法,一次仅考虑
一个数据点。
有时候,我们需要最大化某个函数,这只需要最小化这个函数的负值(相应的梯度函数也
需取负) :
def negate(f):
"""return a function that for any input x returns -f(x)"""
return lambda *args, **kwargs: -f(*args, **kwargs)
def negate_all(f):
"""the same when f returns a list of numbers"""
return lambda *args, **kwargs: [-y for y in f(*args, **kwargs)]
def maximize_batch(target_fn, gradient_fn, theta_0, tolerance=0.000001):
return minimize_batch(negate(target_fn),
negate_all(gradient_fn),
theta_0,
tolerance)

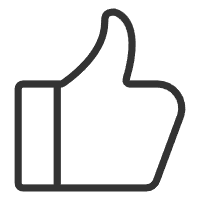
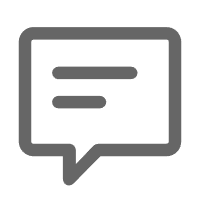
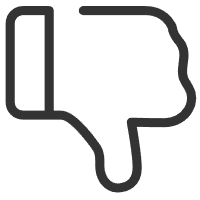
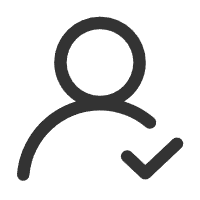
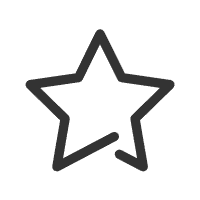
评论(0)
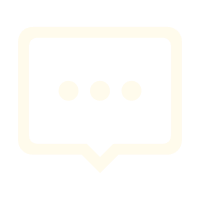

暂无数据
推荐帖子
0条评论
0条评论
0条评论