2021-03-15
阅读量:
1104
若是逻辑回归二分类想画roc曲线第二个参数应该传的是什么代码呢?
样本属于正样本的概率
roc_curve和auc的官方说明教程示例:
from sklearn.metrics import roc_curve, auc # 数据准备 >>> import numpy as np >>> from sklearn import metrics >>> y = np.array([1, 1, 2, 2]) >>> scores = np.array([0.1, 0.4, 0.35, 0.8]) # roc_curve的输入为 # y: 样本标签 # scores: 模型对样本属于正例的概率输出 # pos_label: 标记为正例的标签,本例中标记为2的即为正例 >>> fpr, tpr, thresholds = metrics.roc_curve(y, scores, pos_label=2) # 假阳性率 >>> fpr array([ 0. , 0.5, 0.5, 1. ]) # 真阳性率 >>> tpr array([ 0.5, 0.5, 1. , 1. ]) # 阈值 >>> thresholds array([ 0.8 , 0.4 , 0.35, 0.1 ]) # auc的输入为很简单,就是fpr, tpr值 >>> auc = metrics.auc(fpr, tpr) >>> auc 0.75
调用完roc_curve以后,我们就齐全了绘制ROC曲线的数据。接下来调用plt即可,还是用官方的代码示例一步到底。
import matplotlib.pyplot as plt plt.figure() lw = 2 plt.plot(fpr, tpr, color='darkorange', lw=lw, label='ROC curve (area = %0.2f)' % auc) plt.plot([0, 1], [0, 1], color='navy', lw=lw, linestyle='--') plt.xlim([0.0, 1.0]) plt.ylim([0.0, 1.05]) plt.xlabel('False Positive Rate') plt.ylabel('True Positive Rate') plt.title('Receiver operating characteristic example') plt.legend(loc="lower right") plt.show()

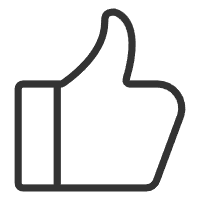
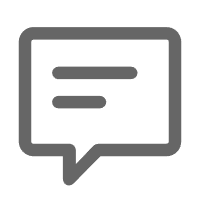
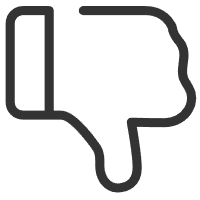
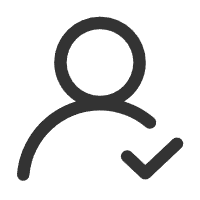
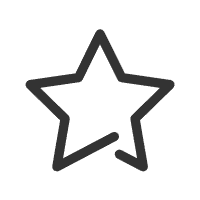
评论(0)
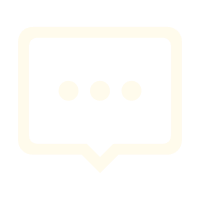

暂无数据
推荐帖子
0条评论
0条评论
0条评论