训练模型
现在,是时候使用我们的数据集训练一些预测模型了。Scikit-learn提供了广泛的机器学习算法,它们具有统一/一致的接口,用于拟合,预测准确度等。
下面给出的示例使用KNN(K最近邻居)分类器。
注意:我们不会详细介绍算法的工作原理,因为我们只想了解它的实现。
现在,请考虑以下示例:
# load the iris dataset as an example
from sklearn.datasets import load_iris
iris = load_iris()
# store the feature matrix (X) and response vector (y)
X = iris.data
y = iris.target
# splitting X and y into training and testing sets
from sklearn.model_selection import train_test_split
X_train, X_test, y_train, y_test = train_test_split(X, y, test_size=0.4, random_state=1)
# training the model on training set
from sklearn.neighbors import KNeighborsClassifier
knn = KNeighborsClassifier(n_neighbors=3)
knn.fit(X_train, y_train)
# making predictions on the testing set
y_pred = knn.predict(X_test)
# comparing actual response values (y_test) with predicted response values (y_pred)
from sklearn import metrics
print("kNN model accuracy:", metrics.accuracy_score(y_test, y_pred))
# making prediction for out of sample data
sample = [[3, 5, 4, 2], [2, 3, 5, 4]]
preds = knn.predict(sample)
pred_species = [iris.target_names[p] for p in preds]
print("Predictions:", pred_species)
# saving the model
from sklearn.externals import joblib
joblib.dump(knn, 'iris_knn.pkl')

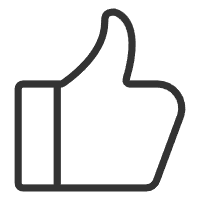
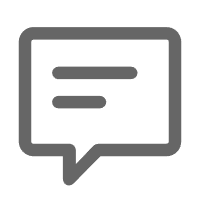
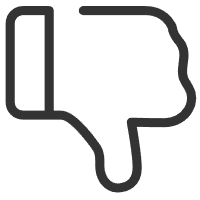
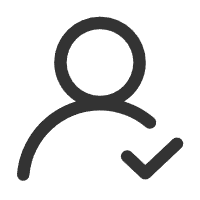
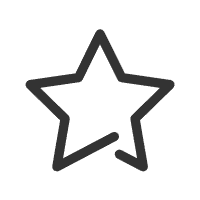
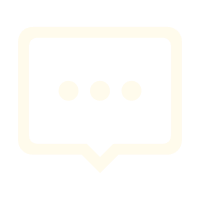

暂无数据